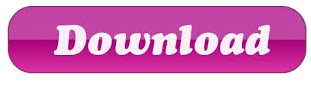
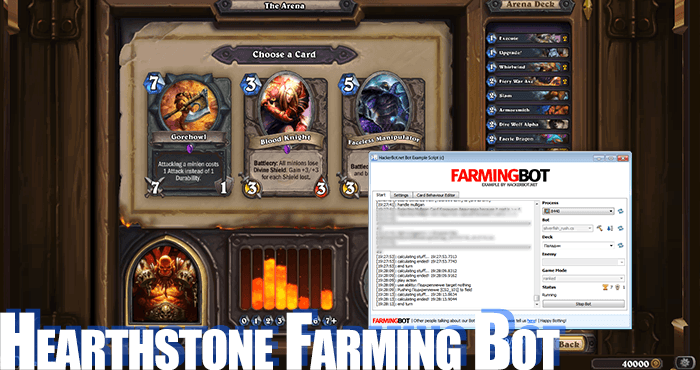
When our extension, Q-attention with Tree Expansion (QTE), replaces standard Q-attention in the Attention-driven Robot Manipulation (ARM) system, we are able to accomplish a larger set of tasks especially on those that suffer from "coarse ambiguity".
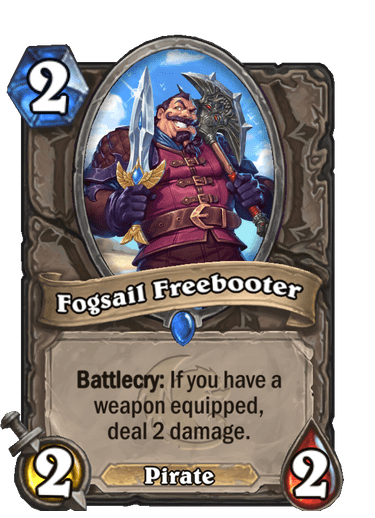
To combat this, we propose to envision Q-attention as a tree that can be expanded and used to accumulate value estimates across the top-k voxels at each Q-attention depth. Although effective, Q-attention suffers from "coarse ambiguity" - when voxelization is significantly coarse, it is not feasible to distinguish similar-looking objects without first inspecting at a finer resolution. Coarse-to-fine Q-attention enables sample-efficient robot manipulation by discretizing the translation space in a coarse-to-fine manner, where the resolution gradually increases at each layer in the hierarchy.
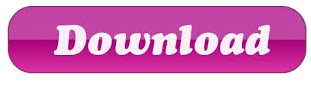